AI-Powered PCs: Rob May discusses the next wave in computing
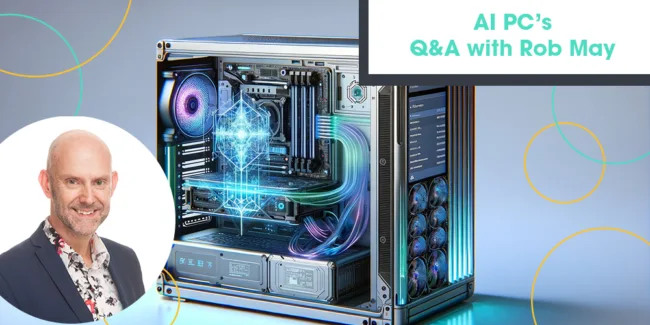
Posted on May 24, 2024 by Louise Howland
Welcome to our technical blog, where we share insights and expertise on a variety of technical topics. This post is part of our ongoing series, specifically aimed at professionals in technical roles, providing in-depth information and practical tips.
In this insightful Q&A, AI expert Rob May delves into the evolving landscape of AI-powered PCs, exploring the integration of cloud and local computing to enhance performance and security. Following Microsoft’s recent announcement about their new AI PCs, Rob sheds light on the importance of CPUs, GPUs, and NPUs in driving this technological advancement.
Is this a new version of Edge computing – computational power from the cloud mixed with local instances?
Yes, I think the movement towards AI-based personal computers (PCs) can be seen as a continuation of edge computing, where computation is done near the data source or the end user instead of depending only on the cloud.
This mixed approach combines the strength of the cloud for intensive tasks and data storage, with the speed and privacy advantages of local processing.
AI PCs demonstrate this by using local hardware acceleration (e.g., GPUs, NPUs) for AI tasks, lowering latency, saving bandwidth, and improving data security by reducing the amount of sensitive data sent to the cloud.
This combination of cloud and edge computing in AI PCs allows a smoother and more effective user experience, supporting a variety of applications from real-time analytics and AI development to gaming and content creation.
I think it marks the next stage in distributed computing, sharing the workload between local devices and the cloud.
Why is the combination of CPU, GPU and NPU so important?
Modern computing tasks, especially in AI and machine learning, require many different computational capabilities that are best met by this combination of CPUs, GPUs, and NPUs.
CPUs, that’s Central Processing Units, they’re the general-purpose processors, and they are good at sequential processing and running the operating system and our conventional applications.
GPUs, that’s Graphics Processing Units, which were originally created for graphics rendering, are very effective at parallel processing, which makes them suitable for the matrix and vector operations that are essential for both AI and Deep Learning.
Then the NPUs, which are Neural Processing Units, are specialised processors specifically for AI tasks, which are designed to efficiently speed up neural network computations whilst maintaining low power consumption.
This combination enables a flexible computing environment where each type of processor can be used for tasks that best fit its advantages, this then leads to significant enhancements in both performance and energy efficiency.
So, for example, a CPU can handle general computing tasks, a GPU can deal with intensive parallel computations for AI model training and inference, whilst an NPU can offer extra acceleration for specific AI algorithms.
This approach is crucial for the creation of complex AI applications, allowing faster processing times, reduced energy consumption, and ultimately the possibility to run increasingly advanced AI models on an ever-increasing number of devices, from PCs to mobile phones and embedded systems.
As it currently stands – beyond Co-pilot – a lot of the current implementations with AI PCs are related to Video conferencing or creative led applications in your view, is the lack of breadth a concern ?
AI implementations in PCs are currently focused on video conferencing and creative applications, and this reflects the early stages of AI integration, targeting areas with high demand and clear benefits from AI enhancement.
Whilst this focus might seem narrow, I think it’s an obvious starting point, using AI where it can make immediate differences, enhancing communication quality and boosting creativity through tools that can edit, generate, or suggest content.
I don’t think the apparent lack of breadth should be a worry but rather seen as a developing landscape. As AI tech becomes more advanced and more accessible, its applications are expected to diversify significantly. The current focus areas serve as a testing ground for its capabilities, for user acceptance, and for the development of the underlying technologies.
Also, these applications drive the improvement of hardware and software ecosystems, which creates the foundations for broader AI integration across various industries and use cases.
As developers and businesses become more familiar with AI’s potential and limitations, new applications will emerge, increasing the presence of AI PCs in sectors like healthcare, education, finance, and beyond.
This evolution follows the path of many technological innovations, where initial applications in high-visibility areas eventually lead to widespread adoption. So, I think the current focus is a step in the broader integration of AI into personal computing, indicating the start of a change in how PCs are used and the kinds of tasks they can do.
How important is having locally operated AI models from a security standpoint?
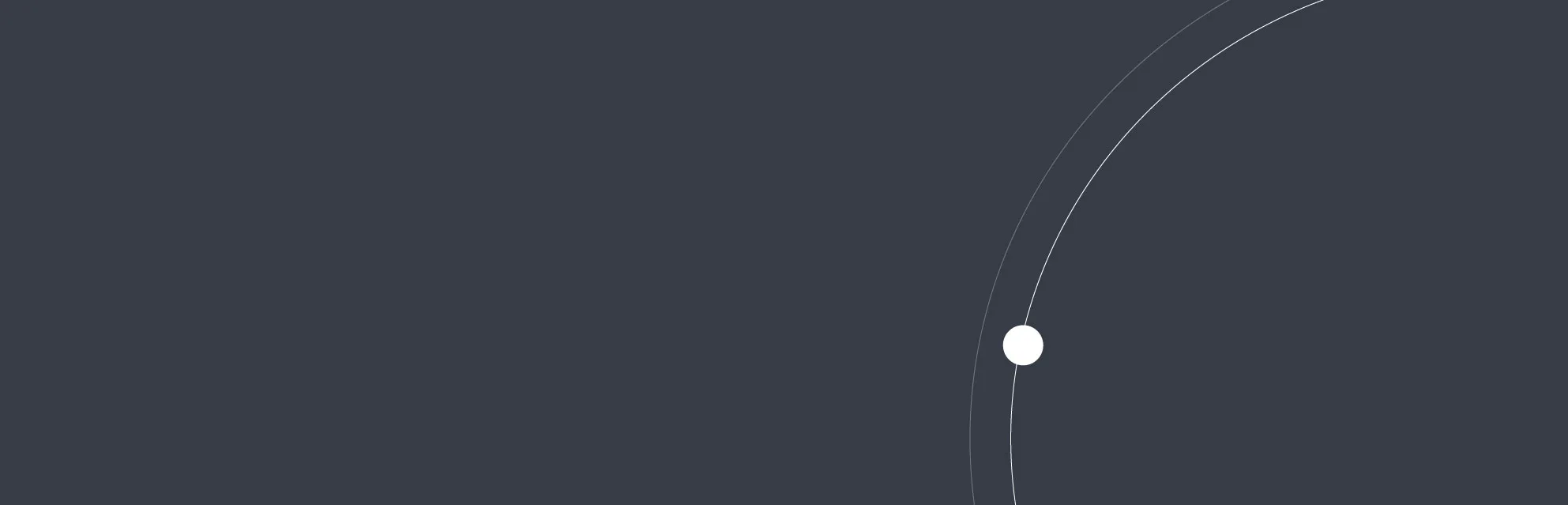
AI prompting techniques (+17 downloadable job role prompt sheets)
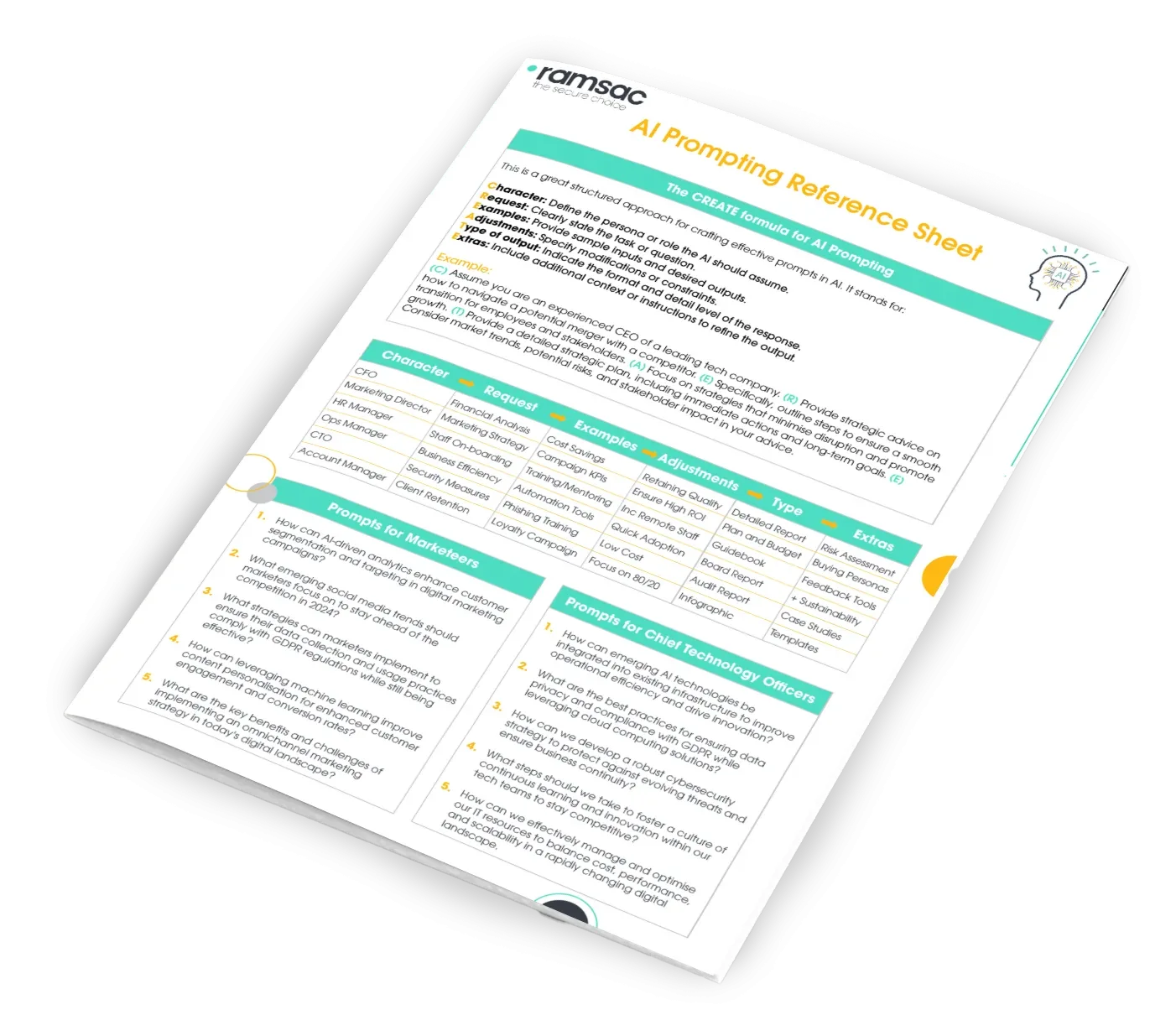
Running AI models on local devices has clear security benefits by addressing issues related to data privacy, integrity, and control.
When AI models run locally rather than in the cloud, sensitive data doesn’t have to be sent over the internet or stored on remote servers. That lowers the chance of data breaches, interception, or unauthorised access, which are all vital considerations in industries dealing with sensitive information like healthcare, finance, and government.
Local processing also makes sure that data stays under the user’s control, following strict data protection regulations such as GDPR. By keeping data on-site or within a local network, organisations can more easily meet legal requirements regarding data residency or sovereignty.
Also, locally operated models can be more resistant to network-related issues, ensuring that essential AI functionalities remain accessible even if cloud services are not available due to connectivity problems or cyberattacks targeting cloud infrastructure.
However, it’s really important to note that local AI models also need strong security measures to protect against local cyber threats, such as malware or physical tampering. The security of all AI systems, involves a comprehensive approach, including secure model training, data encryption, access control, and continuous monitoring for potential threats.
So, while locally operated AI models do offer distinct security benefits, especially in terms of data privacy and regulatory compliance, they also require a balanced security strategy that addresses both local and cloud-based vulnerabilities.
Do you feel that organisations are sufficiently prepared?
No! Most organisations are still learning how to deal with the complexities of using AI in their operations, including understanding the full range of security implications. They don’t yet have a strategy for AI and they certainly haven’t rolled out training to their staff on how to best use these systems.
The fast progress of AI technologies and the changing nature of cybersecurity threats mean that preparedness is not a fixed goal. While some organisations, especially those in sectors like technology, finance, and defence, have made significant progress in securing AI systems and data, the readiness across the board is diverse.
One challenge is the lack of skilled professionals who have both AI and cybersecurity expertise. This talent gap can limit the development and implementation of comprehensive security strategies for AI.
Regulatory compliance is another important area where many organisations are trying to catch up. As governments and industry bodies introduce new regulations to address the privacy and ethical issues of AI, organisations need to adapt their policies and practices, accordingly, often requiring significant effort and resources.
Organisations are also struggling with the balance between using cloud-based AI services for their scalability and flexibility and deploying AI locally to improve security and data privacy. This decision requires a subtle understanding of the trade-offs involved, and the specific needs and risks associated with the organisation’s data and AI applications.
Obviously, some organisations are well-prepared and actively investing in securing their AI operations, but the overall landscape indicates a need for increased awareness, investment, and education.
Continuous learning and adaptation are essential to ensuring that organisations can reap the benefits of AI whilst also reducing the risks.
Any advice on how organisations should approach AI for PC?
There are several factors to consider when looking at AI PCs:
Performance Requirements: Look at the needs of the AI applications to determine the required computational power, memory, and storage. High-performance GPUs or NPUs may be needed for intensive machine learning tasks.
Scalability: Make sure the hardware can handle increasing AI workloads and complexity. Look to ensure that the systems you’re buying are modular or upgradable which will provide flexibility as needs change.
Energy Efficiency: For AI models that run continuously, consider the energy use of the hardware. GPUs or NPUs that are energy-efficient will help control operating costs and obviously reduce the environmental impact.
Latency: For AI applications that need real-time responses, low-latency hardware is essential. Local processing with adequate GPUs/NPUs will reduce latency compared to cloud-based solutions.
Security: Hardware needs to have advanced security features to safeguard sensitive AI data. Including secure boot, hardware-based encryption, and virtualisation support for secure environments.
Compatibility: Confirm the hardware compatibility with AI software and frameworks that you want to use. The hardware should support various platforms and programming models to prepare for future developments.
Cost: Weigh up the cost of high-performance AI hardware against the expected ROI. Budgeting should account for the total cost of ownership, including maintenance and possible upgrades.
Regulatory Compliance: Follow regional regulations regarding data processing, especially if AI applications involve personal or sensitive data.
Choosing AI-compatible hardware will help organisations make the most of AI, improving productivity and maintaining a competitive advantage.
Rob May’s insights highlight the transformative potential of AI PCs, underscoring the need for organisations to strategically prepare for this next phase of personal computing. As AI continues to evolve, embracing these advancements will be crucial for staying competitive and secure in a rapidly changing technological landscape.
Find out how ramsac can help you prepare your organisation for AI, click here.
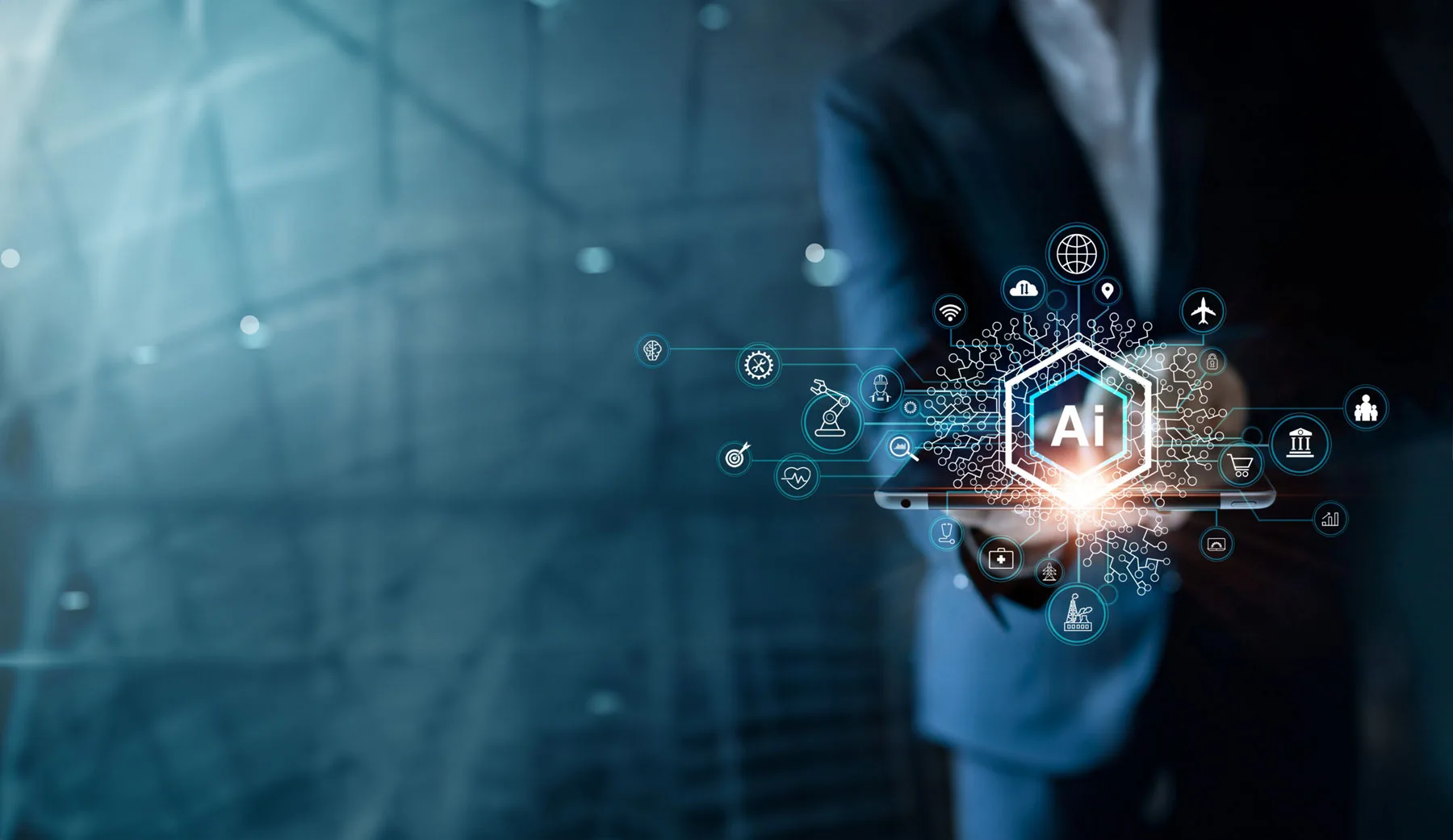
Take the ramsac AI Readiness Assessment
Our AI readiness assessment looks at what your organisation needs to implement AI, and provides you with clear guidance and support to make it happen.