Unlocking the future: Introduction to AI PC’s
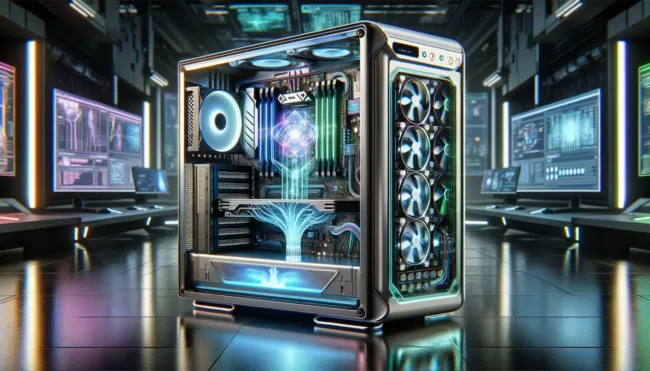
Posted on May 20, 2024 by Rob May
Welcome to our technical blog, where we share insights and expertise on a variety of technical topics. This post is part of our ongoing series, specifically aimed at professionals in technical roles, providing in-depth information and practical tips.
AI PCs are becoming more popular as there is more need for high computing power and efficiency to process large amounts of data, especially for Artificial Intelligence (AI) and Machine Learning (ML) tasks. This has led to the creation of specialised hardware, such as GPUs and NPUs, which are designed for the parallel processing tasks that are common in AI calculations. Moreover, software improvements are aiming to optimise algorithms for these hardware platforms, enhancing efficiency and allowing more complex models to be run on desktop settings. The growth of AI PCs is also supported by the incorporation of AI features in everyday applications, from voice recognition and language translation to content creation and gaming, making AI more available to a wider audience. As the technology progresses, we’re seeing a move towards more energy-efficient designs and the distribution of AI tools, making them accessible to enthusiasts, researchers, and professionals alike.
What is a GPU?
A GPU, or Graphics Processing Unit, is a specialised processor initially designed to accelerate the rendering of 3D graphics and images. However, it has been adapted to perform computation in applications traditionally handled by the Central Processing Unit (CPU), especially those requiring parallel processing power. This makes GPUs highly effective for tasks such as machine learning, video editing, and scientific computations, where handling multiple operations simultaneously is beneficial.
What is a TPU?
A TPU, or Tensor Processing Unit, is a type of Application-Specific Integrated Circuit (ASIC) developed specifically for neural network machine learning. It is tailored for a specific set of computations typically found in deep learning tasks. TPUs are designed to accelerate tensor operations, which are the core operations in neural network calculations, providing high throughput and efficiency. They are primarily used in data centres for AI workloads that require a lot of matrix calculations, such as training and inference for deep learning models.
What is an NPU?
An NPU, or Neural Processing Unit, is a specialised hardware accelerator designed explicitly for neural network computations, which are fundamental to artificial intelligence and machine learning. NPUs are optimised to perform the high-volume, low-power, and parallel computations required for tasks like image recognition, natural language processing, and other AI-driven applications.
NPUs are typically more efficient than general-purpose CPUs for AI tasks because they are tailored to execute the tensor and vector operations that neural networks require. They are similar to GPUs in their ability to handle parallel processing but are often more power-efficient and can be integrated into smaller devices such as smartphones, IoT devices, and edge computing nodes.
By offloading AI processing tasks from the CPU or GPU to an NPU, devices can run AI algorithms faster, more efficiently, and with lower energy consumption, which is crucial for battery-powered and mobile devices where power draw and heat dissipation are significant concerns. This specialised processing is becoming increasingly important as AI and machine learning become more pervasive in various applications and devices.
How does an NPU differ to a TPU?
Both NPUs (Neural Processing Units) and TPUs (Tensor Processing Units) are designed for accelerating machine learning tasks, yet they differ in their design philosophy, deployment, and sometimes in their specific functionalities.
TPUs are a proprietary technology developed by Google, intended for their data centres to accelerate tensor operations primarily in deep learning applications. They’re integrated into Google’s cloud infrastructure and optimised for TensorFlow, a popular machine learning framework. TPUs are designed to provide high throughput for both training and inference of machine learning models, with a particular emphasis on large-scale and complex neural network computations.
NPUs, on the other hand, are a more generic term that can refer to any specialised hardware designed to process neural network-related tasks. Various semiconductor companies produce NPUs, which can be found in a range of devices from smartphones to edge devices. NPUs may not match the raw performance of TPUs, especially in a data centre environment, but they are optimised for power efficiency and quick inference, making them suitable for consumer devices.
In essence, the main differences lie in their use cases and the scale of operations they are designed for. TPUs are tailored for heavy-duty machine learning tasks in cloud and enterprise environments, while NPUs are versatile and intended for on-device AI applications, balancing performance with power efficiency to suit the needs of consumer electronics.
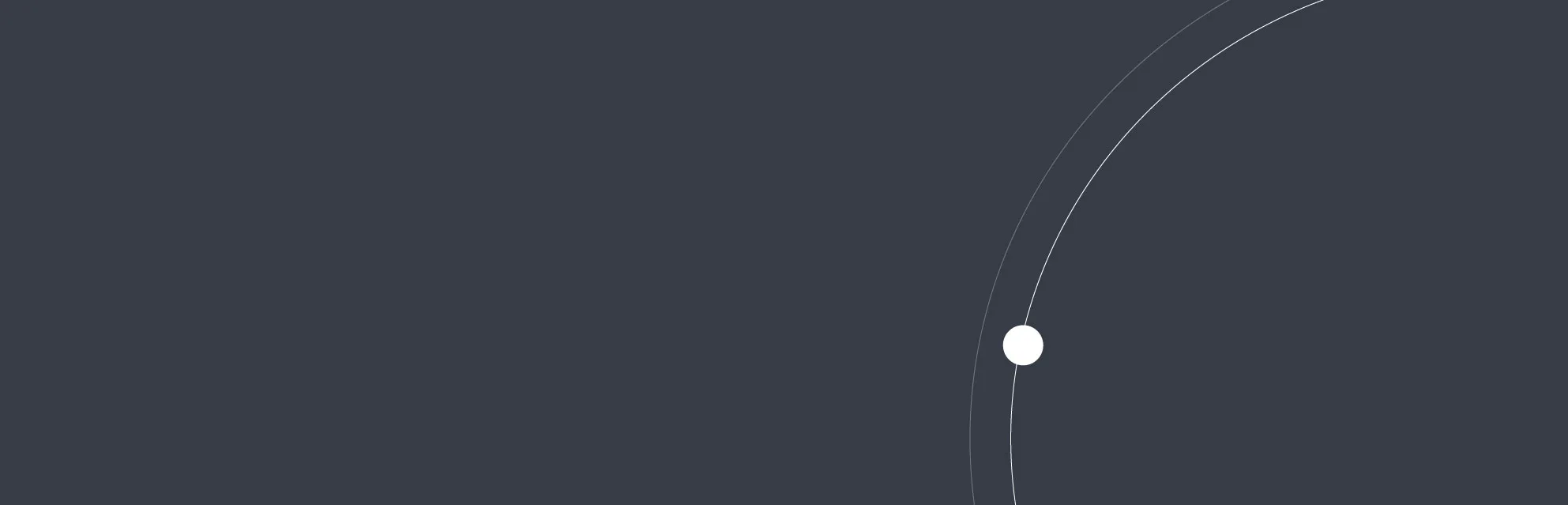
AI Prompting Guide
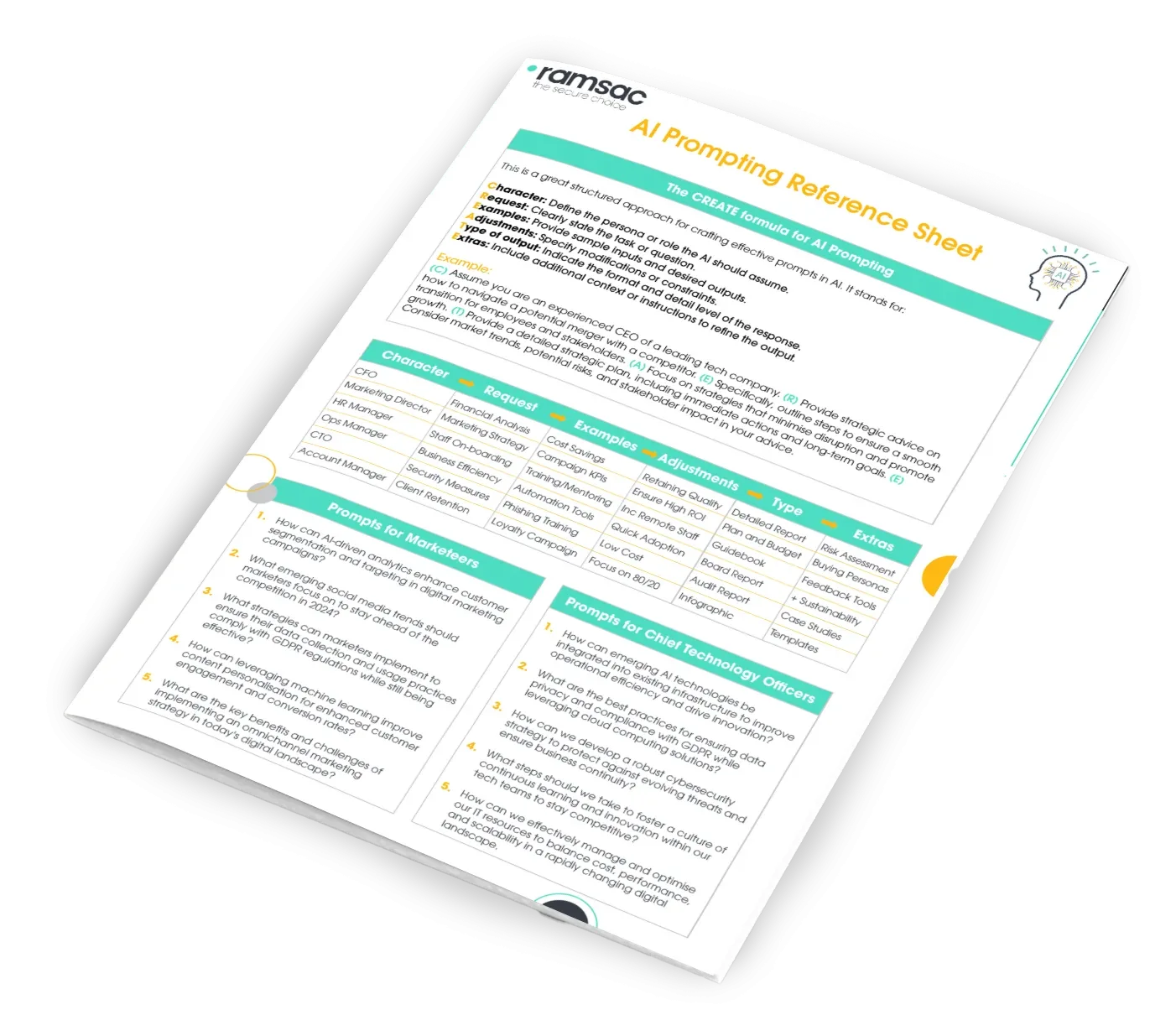
Three types of NPU-enabled AI PCs
There are currently three types of NPU-enabled AI PCs, these are “hardware-enabled AI PCs”, “next-generation AI PCs” and “advanced AI PCs”.
- Hardware-enabled AI PCs will include an NPU offering less than 40 tera operations per second (TOPS) performance, among other things. Looking at the market Qualcomm, Apple, AMD, and Intel are all shipping chips in this category.
- Next-generation AI PCs includes an NPU with 40-60 TOPS performance, and Advanced AI PCs a TOPS of over 60.
- AI-enabled PCs will bring a dramatic acceleration to productivity.
As we delve into the era of AI-powered PCs, it’s clear that these advancements are revolutionising the way we interact with technology. With the increasing demand for high computing power and efficiency, specialised hardware such as GPUs, TPUs, and NPUs are at the forefront of this transformation, enabling complex AI and ML tasks to be executed more efficiently. The integration of AI features into everyday applications, from voice recognition to gaming, is making AI accessible to a broader audience.
The progress in both hardware and software is driving more energy-efficient designs and broader distribution of AI tools, benefiting enthusiasts, researchers, and professionals alike. As AI technology continues to evolve, we can expect even greater enhancements in productivity and innovation, paving the way for a future where AI is seamlessly woven into the fabric of our daily lives.
Find out how ramsac can help you prepare your organisation for AI, click here.
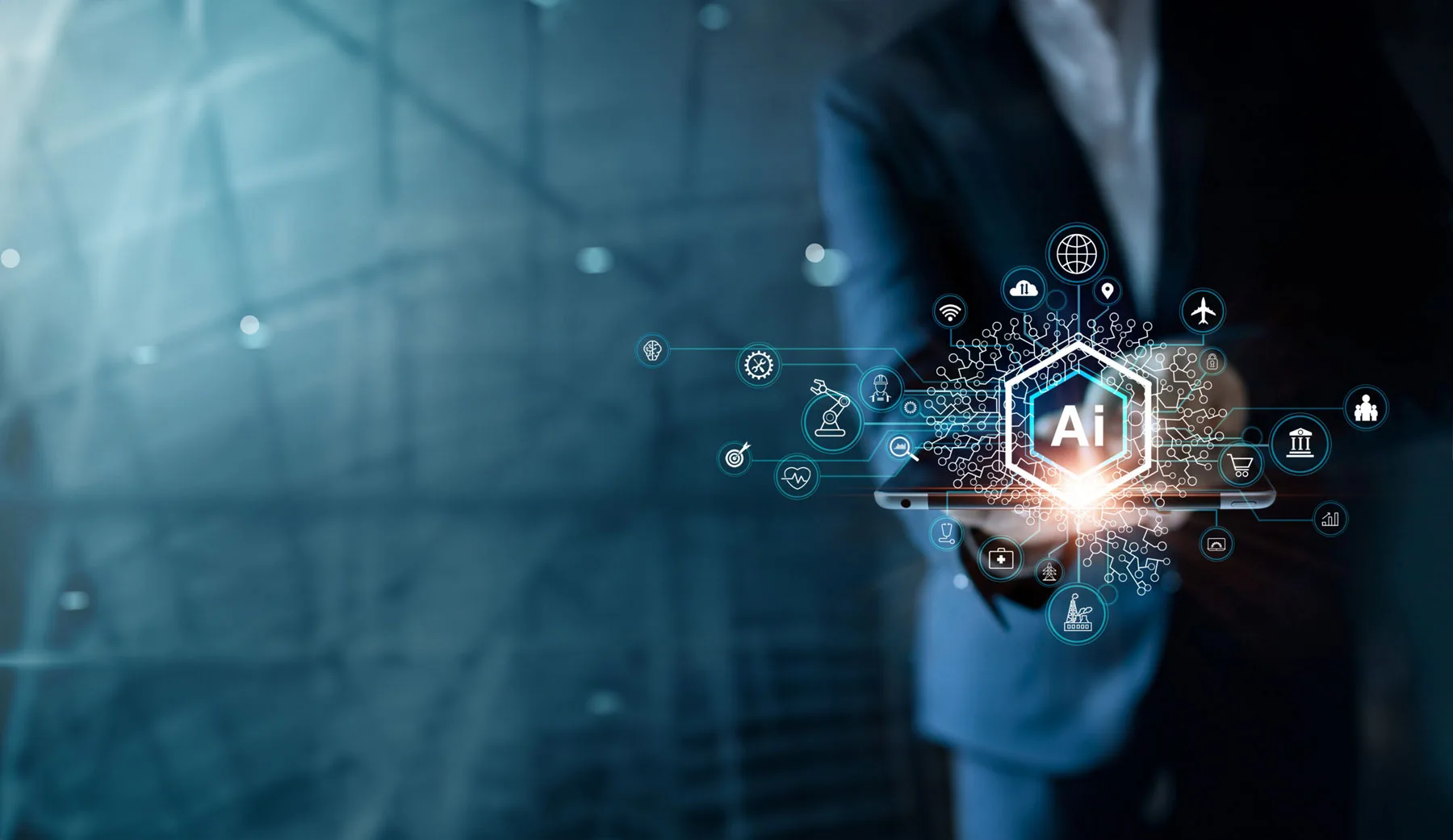
Take the ramsac AI Readiness Assessment
Our AI readiness assessment looks at what your organisation needs to implement AI, and provides you with clear guidance and support to make it happen.